Tackling Socioeconomic Bias in Machine Learning with the Dollar Street Dataset


Despite the meteoric rise of ML, most commercially available datasets only represent a small fraction of humanity, with a skew towards high-income populations. To address this, we co-created the open-access Dollar Street Dataset, alongside our partners at Gapminder, Harvard University, and MLCommons.
By retraining an established CV model on this new, socioeconomically diverse dataset, we improved classification accuracy for items from lower-income households by 50%.
We were also able to raise model performance across all income quartiles to an average success rate of 75%. In this article, we’ll outline how the AI community and business leaders can use the Dollar Street Dataset to combat socioeconomic bias in their algorithms.
The Dollar Street Dataset is a collaborative non-profit project between Swedish NGO Gapminder, international non-profit ML Commons, Harvard University, and machine learning start-up Coactive AI. We are united in our resolve to combat socioeconomic bias – whether in people, algorithms, or social systems.
In brief: algorithmic bias and its impacts
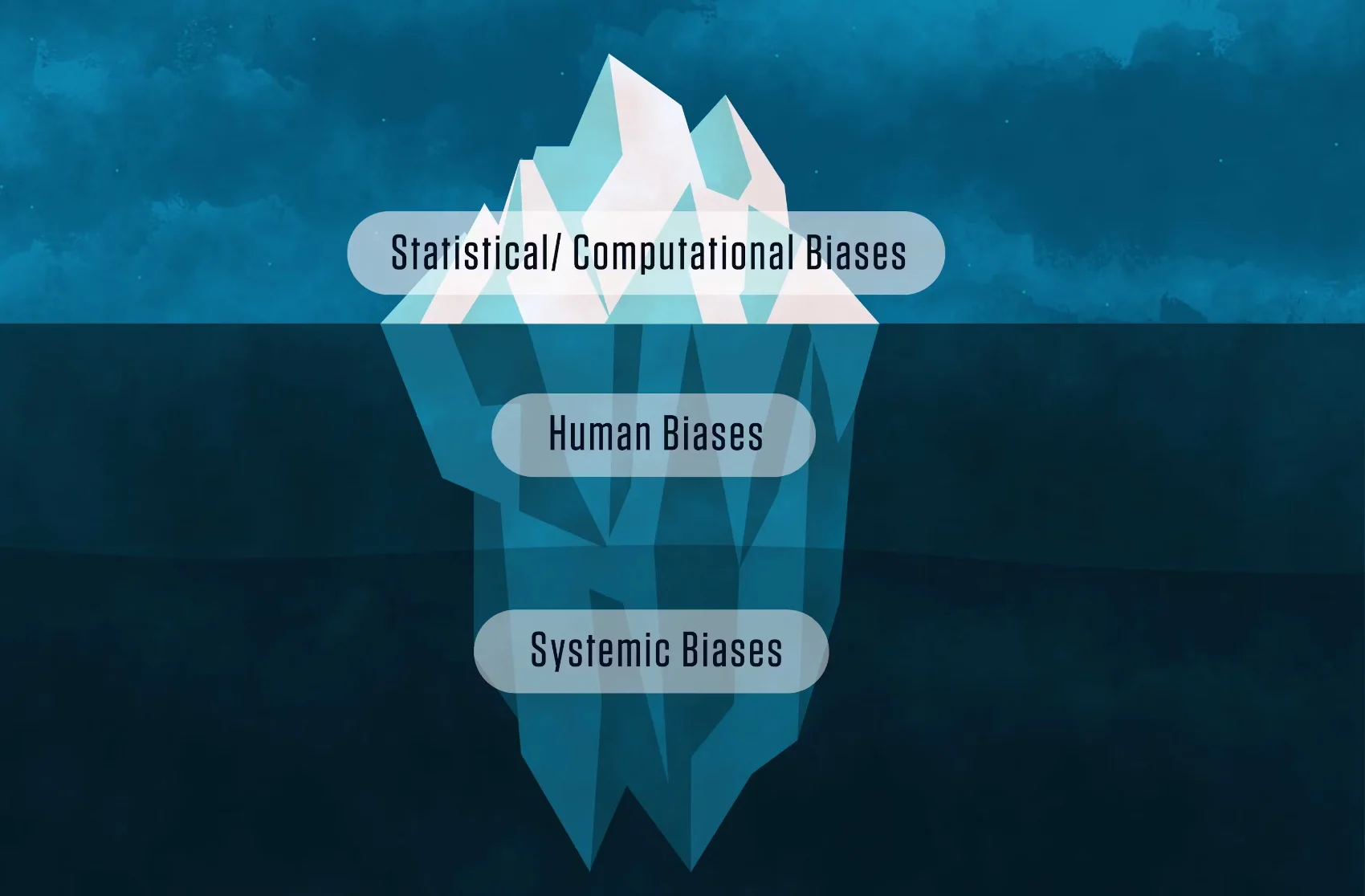
[Image source, image credit: N. Hanacek/NIST]
Algorithmic bias affects people daily, disproportionately harming women and people of color, and widening social inequalities [1, 2]. For instance, industry-leading CV tools misidentify darker-skinned females 1 in 3 times [3], and algorithmic bias in the criminal justice system is leading to wrongful arrests within minority communities [4].
The historic lack of high quality, diverse training datasets is a big causal factor of algorithmic bias [5, 6]. We’ve been teaching our ML models to see the world through a narrow amero/eurocentric lens [7, 8].
“Our own values and desires influence our choices, from the data we choose to collect to the questions we ask. Models are opinions embedded in mathematics.”
― Cathy O'Neil, Weapons of Math Destruction: How Big Data Increases Inequality
To combat algorithmic bias, businesses need to train their ML algorithms on datasets that are representative of all the populations that will be affected by AI deployment. Modern companies and HR teams have learned that inclusive, diverse workforces perform better, and it’s time for the ML community to apply the same wisdom to its training data, especially as their products and services start to impact billions of people across emerging economies and developing countries [9, 10].
How the Dollar Street Dataset can help combat algorithmic bias?
The Dollar Street Dataset is an open-access dataset designed to combat socioeconomic bias. We hope it will inspire and enable ML developers to deliver more socioeconomically-inclusive algorithms and a fairer future for all communities.
The dataset is well-suited to fine-tuning CV models. It holds 38,000+ images of household items with highly detailed demographic tags including location, region, objects, and household income. 63 countries are represented, including communities that don’t yet have internet access, and all metadata has been generated and verified by humans thanks to the painstaking work by Gapminder.
We tested the Dollar Street Dataset on image classification tasks using five of the most widely adopted pre-trained CV models. We found that the classification accuracy of household items was correlated with household income. In fact, we showed that accuracy drops from 57% for high-income homes to just 18% for low-income homes.
However, by using the Dollar Street Dataset to fine-tune one of the pre-trained models, we were able to overcome the model’s original socioeconomic bias and achieve classification accuracy of around 75% across all incomes. In other words, without changing the model itself, we were able to eliminate the algorithmic performance gap between rich and poor households.
How was the Dollar Street Dataset created?
This project is a collaboration between three organizations. It builds on the Dollar Street project by Gapminder, a Swedish non-profit organization. Gapminder rigorously coordinated an international network of professionals and volunteers to collect the necessary data. These people visited hundreds of homes across 63 countries, collecting tens of thousands of images and metadata details, all of which Gapminder manually validated. We believe this is one of the first datasets to have been collected in this way at such a scale.
The data was put into Gapminder’s interactive tool called Dollar Street. The tool helps to educate people about global income distributions and how the quality of life varies geographically (Check out the TED Talk by Gapminder co-founder, Anna Rosling Rönnlund, showcasing the power of these insights). However, this initial tool was designed for educating everyday people. To make the data available to ML practitioners required a different approach, which is where Coactive AI, Harvard University, and ML Commons stepped in.
Coactive AI provided expertise and resources to make the dataset usable for machine learning engineers worldwide. ML Commons and Harvard University undertook the vital responsibility of hosting and maintaining the dataset, and making it permanently available to all under a Creative Commons license (CC-BY).

[Sudnya Diamos, software engineer at CoactiveAI and co-lead author of The Dollar Street Dataset publication, preparing to present her team’s findings at NeurIPS 2022.]
Our organizations each recognize that proper representation is a key antidote to algorithmic bias, and this project is our joint-effort to provide an industry-wide solution and galvanize positive change.
Summary
This project would not have been possible without the incredible efforts of the Gapminder foundation in creating the source data, and the support of MLCommons in making the curated dataset publicly available.
By training machine learning algorithms using diverse datasets such as the Dollar Street Dataset, we can help close the ML performance gap that disproportionately affects underrepresented communities.
At the beginning of this article, we briefly outlined how algorithms can unintentionally be racist, sexist, and classist [11, 12], and that these implicit biases harm vulnerable people the most [13]. On top of the moral obligation of AI practitioners to address this, domestic policy shifts and global market forces are likely to require anti-bias measures and more algorithmic transparency soon [14, 15, 16].
Responsible AI can deliver a fairer, better world for everyone, and it is our hope that the Dollar Street Dataset will catalyze positive change. We ask users of this dataset to adopt forward-thinking, ethical best practices in its deployment.
With this research, we have sought to demonstrate proof of concept. It is our hope that the Dollar Street Dataset will enable commercial and academic AI practitioners to access high-quality, socioeconomically representative training data, and tackle a key source of algorithmic bias. For more details on our methodology, please see our NeurIPS paper.
If you’re a machine learning engineer, we ask that you test it out or help spread the word. The Dollar Street dataset is simple to download and use, and it can be included in most existing computer vision solutions.