DataPerf: the new data-centric standard for progress in machine learning

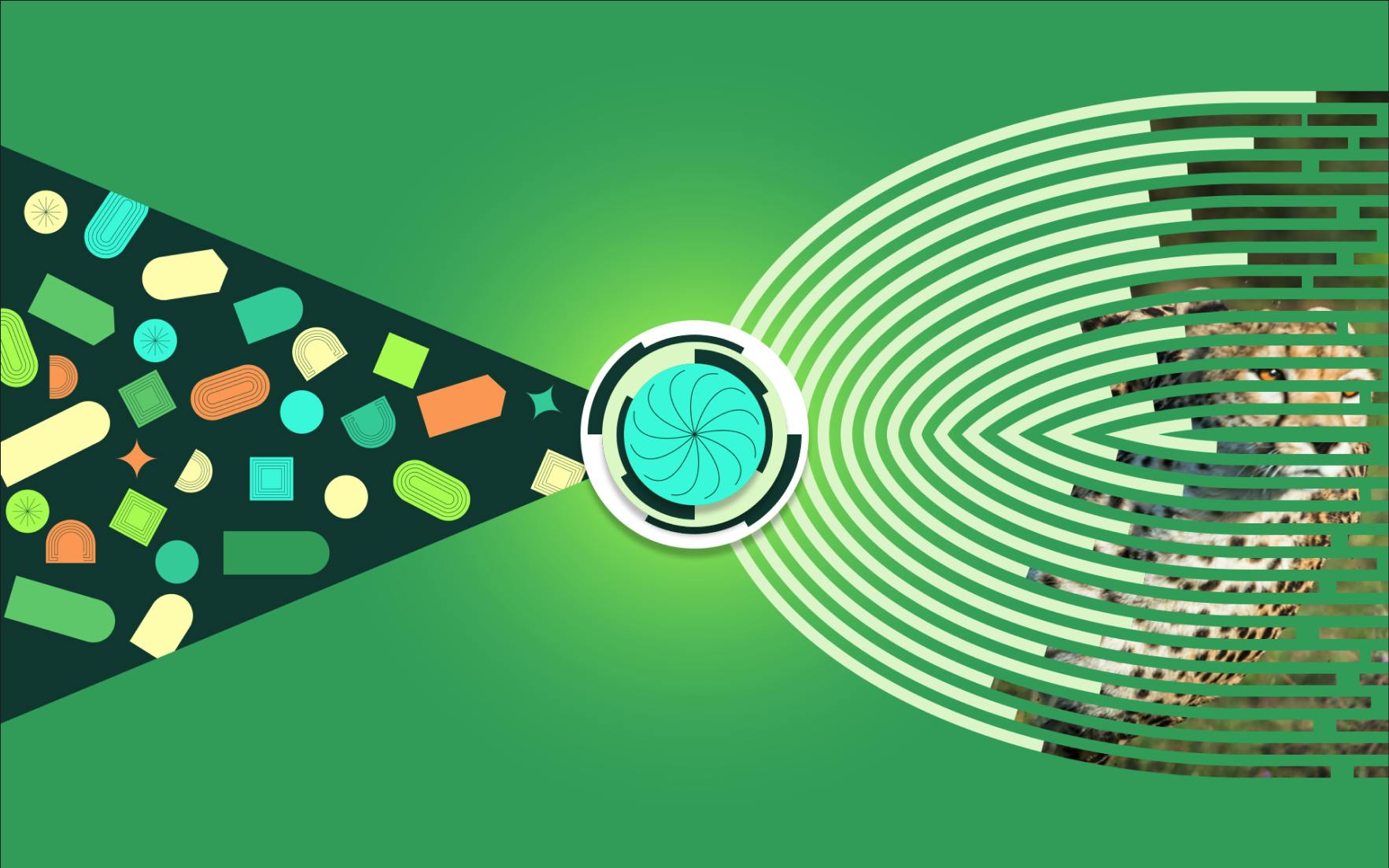
Why does AI perform well in the development sandbox, then fail in real-world production? Because we’ve been training it to ace the test, not preparing it for reality, says Coactive AI co-founder Will Gaviria Rojas.
For AI to live up to the hype, it needs to become reliable in the real world. But how? It’s time to overhaul outdated ML development methods, where algorithms are iterated ad nauseam yet data remains static. Let’s shift our focus to iterating on the data we use to train and test ML models, so that AI can function when it leaves the lab.
In this blog we outline:
- the pitfalls of model-centric development for real-world ML solutions
- how data-centric AI can deliver better real-world results
- DataPerf: the new industry benchmarking tool
- how we’re delivering truly operational AI for businesses
If you think data reigns supreme and you’re serious about deploying ML in the real world, then read on to discover how DataPerf is driving the field towards data-centric AI.
The problem: current industry benchmarks are misleading
As any ML engineer will tell you, there are three core steps to refining a machine learning model: training, testing, and iteration. First, developers will select training data and feed it into their model. Once training is done, they test the model’s performance against industry benchmarks – standardized datasets, like ImageNet, CIFAR10, and OpenImages, that everyone else uses for the same purpose.
The problem is - using static sets of test data is not a reliable way to evaluate a model’s real-world performance. It took fifteen years for the first machine learning tool to reach the coveted and dubious accolade of “human parity”. Modern algorithms can now claim to achieve that in a single year. But these results bear little relation to how an algorithm will perform in the real world. In other words, the test is broken.
Imagine you’re an Olympic coach who exclusively uses treadmills to train athletes. No one would take you seriously if you then claimed your athletes were ready to win a decathlon. Yet, as an industry, this is exactly how we’ve been developing our ML tools: training them narrowly, testing them narrowly, and then watching them flounder upon deployment.
The solution: DataPerf is the new industry benchmark for ML performance
DataPerf is the vital new benchmarking tool for data-centric AI. It’s composed of several interconnected tools developed by Coactive AI, in partnership with ETH Zurich, Google, Harvard University, Landing.AI, Meta, Stanford University, and TU Eindhoven.
It’s essential that companies seeking to deploy machine learning solutions can easily compare the performance of different models — especially for smaller enterprises, who are less likely to have field expertise. DataPerf is raising the bar for the whole ML industry and bringing transparency for clients. By shining a light on best practice, this new benchmark can stimulate faster innovation.
Why engineers should be using data-centric AI methods to improve real-world results
Data-centric AI closes the performance gap by inverting the traditional model-centric ML development process described earlier. Instead of focusing on adjusting your model to raise performance, you focus on feeding it with curated, ever-changing sets of training data. This churn is much more representative of real world scenarios, and deliberately makes it harder for your model to repeat its accuracy.
Imagine going to an archery range to do target practice. With model-centric AI, you might score really high – but that’s because your target is static. With data-centric AI, your targets are constantly moving. As soon as you repeatedly hit the bullseye, the target moves further back. This forces your algorithm to train harder and level up once again. This is how data-centricity yields rapid progress, and delivers machine learning tools that can handle real-world operational complexities.
Businesses need to optimize their training data
Leaving aside the issues with industry benchmarks, businesses have still struggled to operationalize ML tools because of their own data challenges. A business usually either has data that’s too low in quality or too much data to process.
Data-centric AI is the solution to both problems. Focusing on data quality, rather than quantity, can deliver ML tools fit for the real world.
Let’s say your business has a factory which manufactures screws, and you’re training a computer vision tool to detect faults on the production line. Conventionally, you would train the model on a high volume of images, with little regard for their relevance. Instead, you should focus on the quality of your training data.
This data-centric approach also benefits organizations faced with huge data volumes, such as autonomous vehicle manufacturers or UGC platforms. Processing high quantities of data is extremely costly and time consuming. But with data-centric AI, you don’t need to process the entire dataset.
Instead, you should curate subsets of optimized training data which progressively increase in complexity. This way, you can train your ML model efficiently, while making it robust enough to withstand real-world complexities.
There are a few techniques for identifying high quality data. Active learning, curriculum learning, weak supervision, and core set selection are the most common. But new tools are being developed to make this a matter of button clicks for data analysts, rather than a PhD project. If you’re interested in identifying the optimal training data in your data pool, we can help.
Summary
The ultimate goal is to have model-centric and data-centric AI working side by side. But we first need to bring the field of data-centric AI to maturity. To do that, we co-created DataPerf: a new benchmarking system to drive up the performance of machine learning tools in real-world contexts.
The key take-homes for ML practitioners and businesses looking to operationalize AI are:
- Legacy training methods for ML are limiting its real world capabilities, and outdated benchmarking tools are masking its shortcomings
- ML engineers should embrace a data-centric AI approach; stop focusing on model iteration and instead prioritize using high-quality, progressively complex subsets of training data
- DataPerf is the new industry benchmark that will allow ML developers and users to more transparently compare the likely real-world performance of new models
If you want to be part of an ML industry that delivers the dream of operational AI, then you need a new benchmarking system. You need DataPerf.
CoactiveAI is deeply grateful for the support and expertise provided by the co-creators of DataPerf, including ETH Zurich, Google, Harvard University, Landing.AI, Meta, Stanford University, and TU Eindhoven.
Want to learn more about how Coactive can help your organization leverage image and video data? Request a demo today, or reach out to us at info@coactive.ai.
Coactive AI is the industry-leader in data-centric machine learning. We’re the best-in-class solution for analyzing unstructured image data. To request a product demo, get in touch. Or if you’re looking to help build the future, check out our job openings.