Introducing: advanced video analytics using a Multimodal Application Platform (MAP)


Despite sitting on a wealth of video and image content, most organizations still can't answer basic questions about what's actually in their media library and what they can do with it. This applies at both the individual asset level and the overview level. Without visual semantic analysis, or the ability to combine this with audience engagement metrics, companies struggle to leverage data-driven content strategies and extract the full value from their content.
AI-powered Multimodal Application Platforms (MAPs) are changing this. By combining visual content analysis, metadata enrichment, and powerful data analysis tools, companies can get nuanced and customizable analytic insights from their content archives.
Here we’ll explore how MAPs are enabling new possibilities in video analytics – and in turn, new possibilities for content strategy, brand safety, and audience engagement.
Why do analytics matter?
Media and retail businesses with large content archives have three key problems when it comes to analytics:
- Content understanding: limited insight into what’s in their images, videos, and libraries – this reduces asset utilization
- Data integration: no practical way to compare content insights with other data (e.g. engagement metrics) – this reduces strategic insight
- Downstream dependencies: limited analytics reduce businesses’ abilities to optimize future content, personalize recommendations, and moderate unsafe content – increasing cost and risk
For instance, a business might want to know a) how many videos of basketball player Lebron James are on their platform, and b) whether the ones of him shooting hoops or celebrating are more popular. With traditional analytic methods, this kind of inquiry has been speculative at best, and an obstacle to new revenue streams.
Analytics also present brands with a huge opportunity to improve trust and safety. For instance, detecting harmful content nested within seemingly safe videos - like inappropriate imagery spliced into children's content or subtle hate symbols in user-generated posts. Automating this with accuracy and agility to emerging risks requires adaptive, modern analytic tools.
How a MAP transforms content analytics
AI-powered analytics help companies overcome the barrier of scale when handling millions or even billions of images and videos at a time.
Core MAP analytic capabilities:
- Visual content analysis (e.g. mood detection) with confidence scoring
- Scene detection, keyframe analysis, and cut-sheet enablement
- Cross-referencing content insights with engagement data*
- Trend detection through SQL queries
An AI-powered Multimodal Application Platform enables a company to streamline its entire content discovery, analytic, and strategic capabilities – improving its competitive advantage.
*A side note on metadata: Traditional analytic tools have been limited by low-quality underlying metadata. Platforms equipped with multimodal AI can solve this upstream problem through efficient metadata enrichment for visual assets – allowing underlying success factors to be analyzed downstream.
Let’s explore three key use cases for advanced video analytics, powered by AI.
Improving content discovery & recommendations
Example 1: a streaming platform wants to automate content referrals for a specific campaign
Say a streaming platform or broadcaster wants to compile shows for a “Holiday favorites” promotion in the US. With a Multimodal Application Platform, they can leverage AI tools across their content library to enable this.
Firstly, they would ensure all videos are enriched with metadata – ideally using a flexible method like Dynamic Tags. Using these initial tags, the AI will then detect the moods and genres present in each keyframe (moments where the scene changes significantly), and generate additional metadata. These enabling steps take a matter of minutes.
Using SQL, users can then apply these tags (e.g. “holiday,” “family-friendly,” “winter setting” etc.) across their entire dataset, specifying confidence thresholds for greater precision. E.g. if the promotion is targeting a parent’s streaming profile, for instance, the business might set “family friendly” at 80% confidence, but only require 60% confidence for “winter setting”.
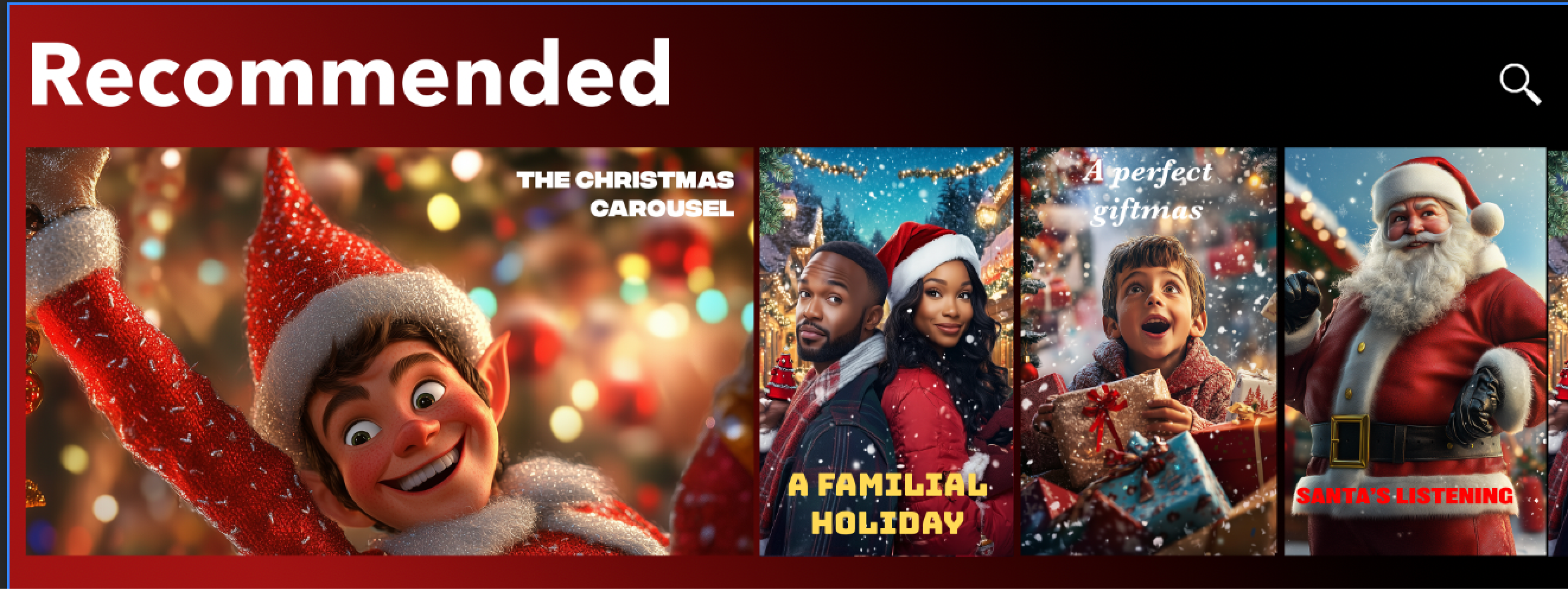
After the promotion period, the streaming platform could cross-reference the semantic visual metadata with viewer engagement data, and examine which content types performed best. They could then carry these insights into the next campaign, optimizing their use of labels, confidence scoring, and weighting, and delivering even better recommendations for viewers.
“Multimodal AI lets you combine different types of inputs to get more precise insights. For instance, for a winter holiday campaign, if 'family' is the tag but you’re specifically looking for families at the dinner table, you can provide visual keyframe examples to guide the model in identifying those moments. Similarly, for identifying images of "winter settings," you could provide a text prompt like 'cars covered in snow' to surface images that reflect snowy winter settings, ensuring your analysis aligns with the exact themes you need.”
– Caitlin Haugh, Product Manager at Coactive AI
Example 2: a UGC platform needs to analyze trends
A platform focused on User Generated Content (UGC) also needs to be able to identify trending topics and recommend content accordingly. For instance, they might analyze the visual content of their top-performing videos from the past quarter and find that those tagged as "motivational speeches" or "cooking tips" are trending sharply upward in engagement. By identifying popular themes, the platform can create specialized discovery channels or playlists, like “Inspiring Moments” or “Cooking Hacks”, capitalizing on new trends and delighting their audiences.
Automating content moderation
Content moderation is a huge challenge for businesses handling large volumes of unstructured video and image data. User Generated Content is growing exponentially, and manual moderation isn’t scalable.
For instance, a platform may need to respond to a new viral trend that’s causing unintended harm. In 2021, TikTok banned videos of the “milk crate challenge”, where people would create them climb unstable piles of plastic bottles, because of a spike in associated injuries. However, they relied on the text hashtag #MilkCrateChallenges to identify and remove the posts. In other words, they were only able to crack down on that instance because users had manually tagged the offending content for them. In most instances, harmful content isn’t labeled so neatly, meaning platforms can’t rely on hashtags to block images and videos before the UGC reaches trusting audiences.
The key unlock is having a system that can understand the contents of visual assets directly. For instance, we helped Fandom, the world’s largest fan platform, reduce their manual moderation hours by 75% across millions of monthly images. Now they’re able to make highly accurate trust and safety decisions within seconds, rather than hours or days.
Multimodal AI can understand the contents of a photo or video in seconds, generate metadata tags that enable cross-platform analysis, and provide confidence scores that enable customized automations for screening purposes. A MAP combines those features in a single place, making the workflow much easier to automate.
Combining data sources to optimize content strategies
Content creators and licensors alike want to be able to recommend the most engaging materials for their client’s audience – and this requires advanced data insights.
For instance, Apple TV’s recent movie “Wolfs” intentionally reunited Brad Pitt and George Clooney in a familiar comedy heist format – i.e. a data-driven commissioning decision with three reliable data points. The right celebrity combination + genre + proof of historic audience engagement = the platform’s most-viewed film debut ever.
AI analytics can help content creators and licensing businesses to unlock hidden performance insights from visual assets, and create winning content strategies for their target audiences.
For instance, a major advertising agency used Coactive to do a sentiment analysis on their historic Super Bowl ads. They were able to label videos as being “uplifting”, “dramatic”, “comedic”, etc. By combining this new visual semantic data with existing campaign success metrics, they were able to train an internal data model that predicts how a new ad will perform. This has enabled them to enhance their advisory services, increasing their competitive edge.
Introducing content analytics with Coactive
The Coactive Multimodal Application Platform allows you to do multiple heavy lifts in one place – like AI-powered search, metadata enrichment, and analytics.
Our analytics features bring new levels of insight to your visual assets, informing your future content strategies:
- Multimodal querying for video analytics at scale: means you can ask “big” questions and the platform will search across your whole media library (image, video, and audio assets together) to identify trends.
- SQL capabilities in the UI: Run custom SQL queries within the Coactive UI to uncover insights about your visual assets, including tag frequency and content trends to enable highly customized analytics.
- Scene detection & keyframe analysis: identify and label significant moments within videos to support segment-based discovery, editing, and downstream analytics. Super efficient with Coactive Intelligent Sampling.
- Visual semantic understanding: leverage AI to understand the actual contents of your visual assets (e.g. moods, actions, themes, logos). This can enable unlock insights into the composition of your library, and support automation of features like content moderation and referral engines.
- Dynamic tagging and metadata enrichment: automatically generate detailed tags and metadata for your media assets, enabling better organization, discovery, and analysis.
- Prepare visual data for your analytics environment: data can be easily exported or imported to enable cross-referencing with other metrics like audience engagement. Whether your team uses a business intelligence tool, cloud data warehouse, or spreadsheet, insights from Coactive can be made available wherever your team does analytics.
- Analytic visualizations: view the semantic makeup of your content library in tables and graphs. Preview videos with their associated data displayed alongside. These custom queries can be viewed inside the Coactive UI – or if you use our API, they’ll appear within your team’s existing interface.
This GIF example shows a Coactive user analyzing their video library for assets relevant to a winter holiday season in the US:
In the GIF above, the Coactive user:
- Enriches the videos with metadata (creating a Dynamic Tags category for various holiday types).
- Performs a custom SQL query to retrieve relevant videos, ranked by confidence scores, across three tags: “Christmas”, “Family”, and “Dinner”
- Toggles between the result formats, switching from the results table to the associated video previews, for easy visual confirmation of result relevance
- Exports the analysis as a CSV file – enabling the user to take the visual content data to their BI tool or equivalent and cross-reference it against performance metrics for the same assets, unlocking new insights
With Coactive, you can understand rapidly changing audience preferences and trends, and derive actionable insights from massive volumes of unstructured data.
Ready for a product demo? Get in touch today.