Introducing AI-powered metadata enrichment – for better content discovery

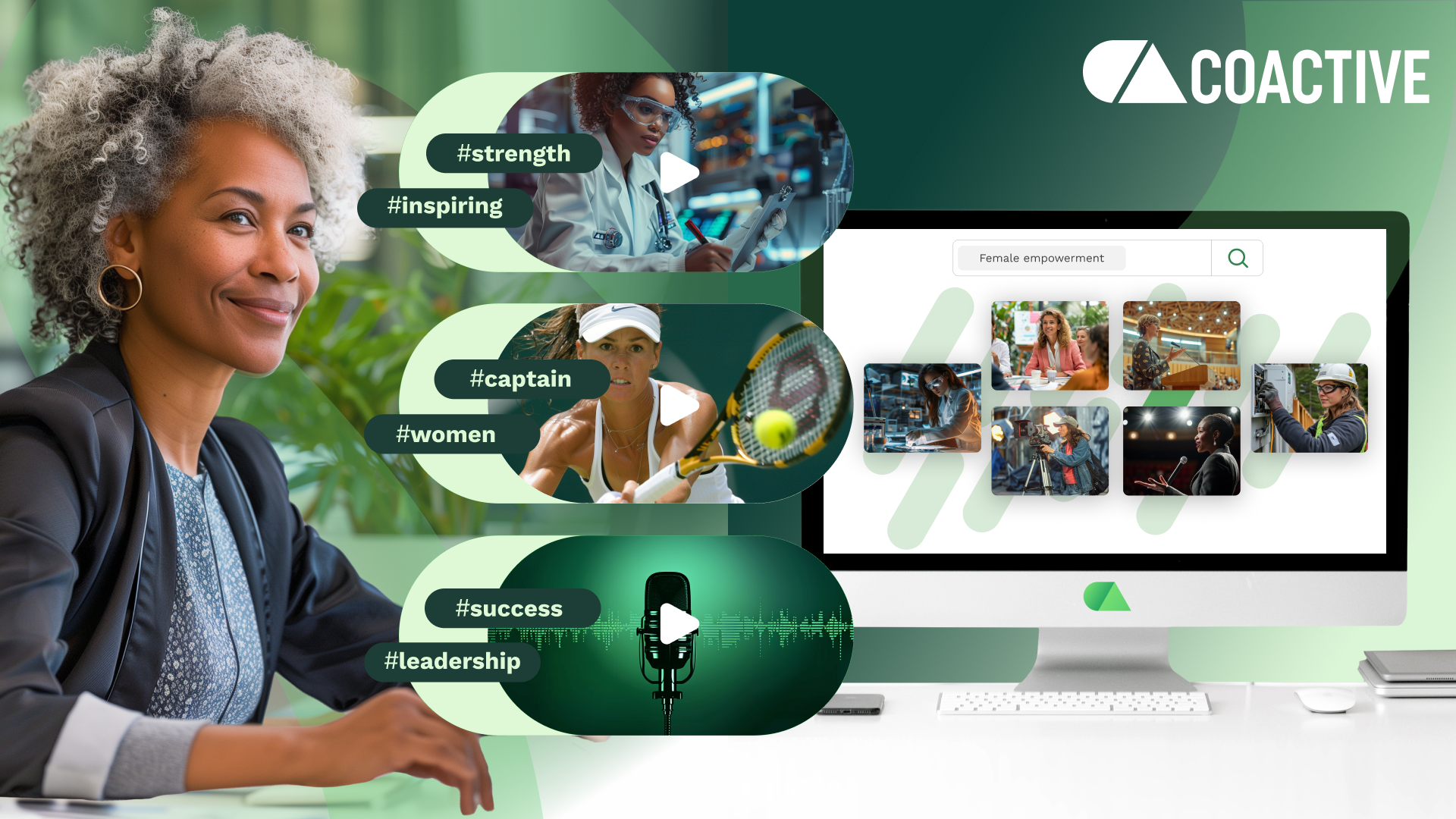
Media businesses have a content discovery problem. When it comes to searching their archives, finding relevant assets is so hard that many resort to recreating photos and videos from scratch. Solving this requires understanding and tackling the root cause – poor metadata.
Traditional search has always relied on keyword tags/labels. This is because legacy search tools can’t “see” images or video – they can only read text. By adding keyword tags (aka metadata) to images and videos, it was possible to make assets discoverable to text-based search tools. So what’s the problem?
Whether you were searching on Google or trying to find a file on your computer, success depended on how well you or someone else had labeled the content. For companies with massive libraries of visual content – think retail product images, news footage, or real estate listings – this is a major problem.
Bad metadata means bad searches. When valuable visual content is poorly labeled, it becomes too hard to find. The advent of multimodal AI is finally changing that – offering businesses a way to make unstructured visual content usable.
Why traditional metadata falls short
Visual content is more important than ever. Whether it’s user generated content in Instagram Reels, product images on Facebook, or TikTok shorts, visual assets drive purchasing behaviors and user action. But with conventional methods, images, videos, and audio are expensive to store and difficult to find.
For example, say you want to find a photo of a family wearing the fall 2024 clothing line. You’d better hope the photographer saved it as something other than 00239898.PNG. If they did manually add labels, are those tags detailed enough for the image to surface in your search? Or will the poorly defined metadata leave your media users drowning in irrelevant results?
"Every minute your team spends manually tagging content is a minute they could have spent generating revenue in better ways. Metadata enrichment through Multimodal AI liberates your team to focus on the value-add parts of the content pipeline, like content creation and analytics."
– Suzanne Singer, Customer Operations Lead at Coactive AI
Manual metadata creation (aka content enrichment) is expensive to produce, with human operatives taking hours to apply a handful of labels to a few hundred images. For companies with millions, if not billions, of visual assets, this approach can never scale.
Three layers of metadata enrichment that power modern content discovery
Search, or content discovery, is how most of your media archive users will experience the benefits of AI. But the foundations of this success rely on scalable, high quality metadata enrichment.

Modern metadata enrichment has three layers. Each layer enables the next, allowing users to unlock more powerful search outcomes.
1. Traditional keywords and static tags
These form the historical foundations of search – but they reveal their limitations quickly. Creation is expensive and highly manual. Legacy keywords excel at basic categorization but fail to capture context or nuance. For instance, a product image tagged simply as "footwear" or "red" misses crucial details that drive real-world queries, such as user demographics, locations, lighting, mood etc. This lack of detail causes a performance gap in downstream search tools.
2. AI-enhanced tagging (Dynamic Tags)
This is nuanced metadata enrichment at scale, and has only become possible with the advent of multimodal AI. Instead of manually entering each label, users can now bulk upload their chosen taxonomy (usually a list of keywords and phrases), and leverage machine learning tools. The AI “reads” your image and video files directly, and applies the relevant metadata labels. This also solves the challenge of metadata becoming outdated, as updating tags is similarly efficient.
3. Customization (Concepts)
Most businesses rely on unique terminology and concepts in their operations or product suite. But these rarely overlap with industry-standard keyword lists, on which mainstream machine learning models are trained. Teaching a model to associate complex and diverse traits like moods, events, under a single search time term, hasn’t been historically scalable. It required businesses to hire technical specialists and invest heavily in computation and fine-tuning.
This is no longer the case. With multimodal AI, it only takes a couple of minutes to provide examples (e.g. images, videos, audio) and train the algorithm to associate chosen assets with your niche search term. With the model trained, you can then apply the new label across all relevant assets in your library. All at the speed and scale of AI.
Your team’s ability to discover content rests on these three layers. The better your ability to generate, update, and customize your metadata, the better your search outcomes.
The enabler: Multimodal AI for content enrichment
As stated, AI-powered content enrichment offers clear advantages when it comes to the speed and scale of your metadata generation. But improving content discoverability requires a drastic improvement in the quality of that metadata, too. The secret sauce? Multimodality.
Multimodal AI is a breakthrough for content enrichment, as it can process multiple types of input simultaneously – just as humans do.
“When we watch videos, humans naturally integrate visual scenes, spoken words, and semantic context. Traditional content discovery tools can’t do that. This has meant there’s been a huge gap between a person’s understanding of visual content versus a machine’s. Multimodal AI changes that. Users can now get human-levels of understanding from the platforms they depend on – transforming business capabilities.”
– Will Gaviria Rojas, co-founder and Field CTO at Coactive AI
By integrating data from different sources like images, text, and audio – in a single process – multimodal AI offers profoundly deeper insights into the actual content of a photo or video than traditional tagging allows. In combining these inputs, multimodal AI enhances the platform’s accuracy and contextual understanding of the content, interpreting not just visual elements but their relationships with dialogue, text, and tone.
For businesses with vast or growing media archives, and an urgent need to improve content discoverability, this is a game-changer. Enrichment is now fast, scalable, more detailed, and customizable.
“Fandom reported a 74% decrease in weekly manual labeling hours after switching to Coactive.”
The results are felt powerfully downstream. Search and analytics operations are suddenly infused with a wealth of information, which manifests as super relevant results and insights for media library users.
Use cases
Running a marketing campaign with a "female empowerment" message
A media network’s ad teams needed to find visual examples of female empowerment for a high profile ad campaign. But historic metadata gaps made relevant content invisible to legacy search tools.
The network used Coactive to identify “female empowerment” assets. To do this, they created reference points across media types. E.g. photos of women speaking at podiums, videos of female athletes, and audio that featured terms like strength, leadership, and equality.
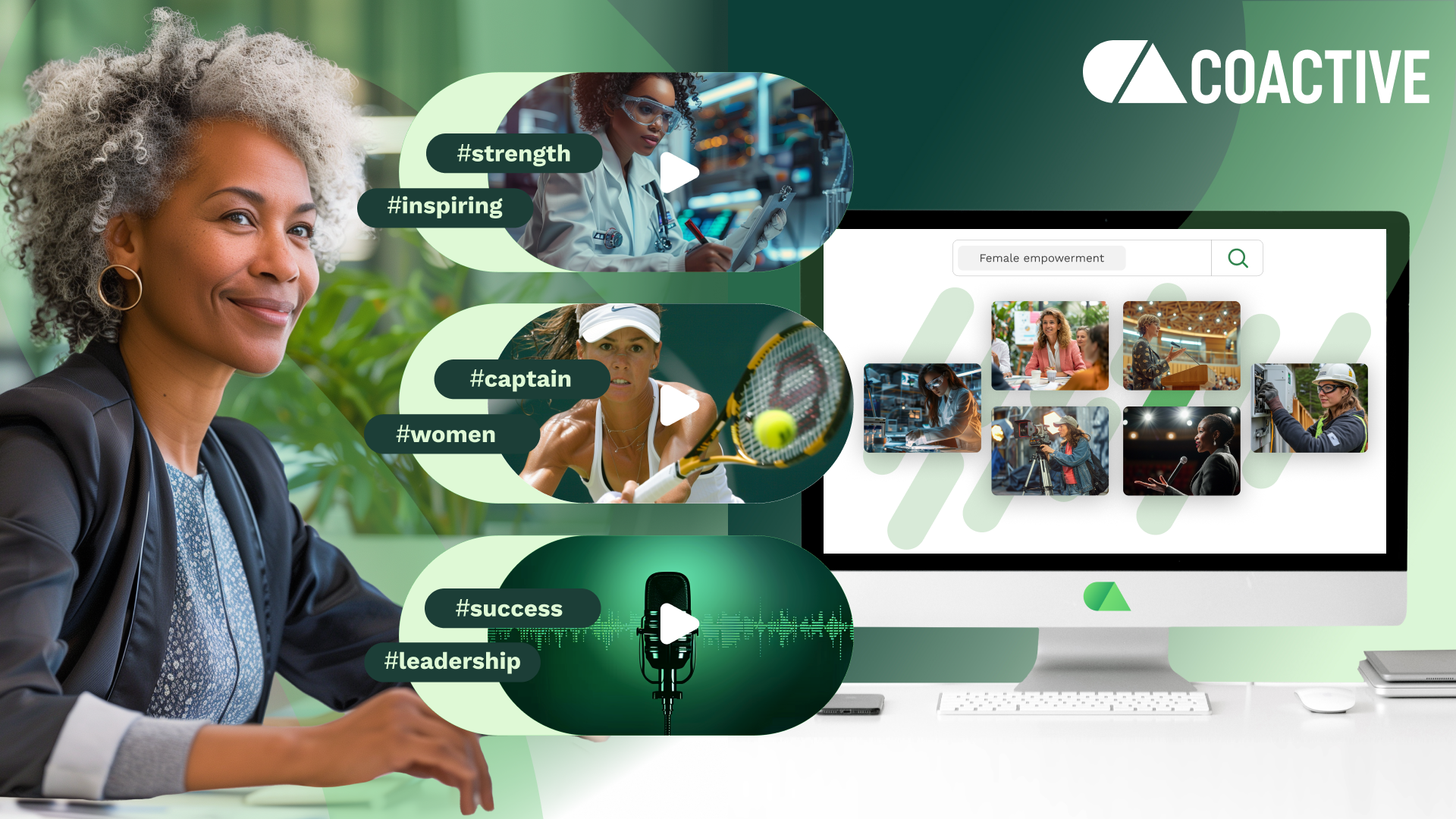
By leveraging the power of multimodal AI – searching with a range of inputs like text, image, and audio – Coactive surfaced highly relevant images and videos pertaining to different aspects of female empowerment. As a result, the client quickly had a range of style options to choose from, and avoided the need to create new assets from scratch.
Finding nuanced trends in visual content
A major casual clothing retailer wants customers to find “athleisure” items on their website. To do this, they need to find a way to efficiently and accurately create and apply that keyword label to all relevant assets in their media archive.
Using Coactive, the retailer could create a custom tag (a “concept”) – efficiently teaching the AI model what they meant by “athleisure”. This can be done using image-to-image search. The user simply uploads photos of people wearing yoga pants, sports hoodies, etc., and tags them as “athleisure”. It only takes a couple of minutes of yes-no clicks to fine-tune the search results, and the concept is understood by the active learning engine, and can be applied across their library.
With metadata enrichment powered by multimodal AI, retailers can keep pace with consumer trends and provide highly relevant search results across their catalogs.
Seizing viral content moments like “Brat Summer”
When content goes viral, businesses scramble to find similar assets in their media archives to capitalize on the moment. But if your media only contains traditional, static keywords, then your search tools won’t know which of your assets are relevant.
Using Coactive's “Concepts” feature, you can quickly train the platform to recognize which of your existing assets align with the new viral trend.
Say a business wants to run a campaign built on the “Brat Summer” spirit. To do this, a user would simply upload images, videos, and audio that encapsulate that highly diverse zeitgeist. For instance, training the platform to look out for the iconic lime green, the Brat font, people dancing to “Apple” by Charlie XCX, or US teens posting grungy fashion outfits, unfiltered photos, and rebellious body language in defiance of previous “Insta-perfect” styles.
The user then provides some quick yes-no feedback to the platform, and the model is trained. Now the business can search across its entire archive and easily surface useful content.
How Content Enrichment Works on the Coactive Platform

Coactive makes powerful AI accessible through an intuitive three-step process:
- Upload your keyword taxonomy → Coactive generates your dynamic tags (metadata)
- Refine your results → Use simple text and image prompts to get quick clarity
- Go niche → Teach the platform your unique idea or “concept” using text, image, or audio prompts
Coactive’s Dynamic Tags feature gives you the power to generate metadata for commonly recognized terms, at the speed and scale of AI. Unlike conventional static metadata, dynamic tags can be easily refreshed at any time. Just upload your keywords, and Coactive will detect and label the relevant assets – making them discoverable to your search teams.
“Dynamic tags can represent a range of topics that can be visually classified, like designer brands, celebrities, and moods. The Coactive Platform makes it easier than ever to organize, filter, and analyze your content quickly and efficiently.”
– Caitlin Haugh, Product Manager at Coactive AI
Custom metadata is also now possible, with Coactive Concepts. Say you’ve used Coactive to populate your archive with Dynamic Tags, and you’re now able to correctly identify all videos containing sneakers. Now you want to differentiate between sneaker brands like Air Jordans vs Air Force 1s?
Simply create a “Concept” by providing a brief written definition in the web application, and labeling around twenty example assets. This labeling can be done via text, image, or video searching, and training is speedy via simple yes-no reinforcement.
Bonus: the Coactive platform is also model agnostic. This is significant because all foundational models are trained differently, and some will perform better than others in given areas. Using a model agnostic platform like Coactive derisks your business from a scenario where your chosen foundational model underperforms or becomes unavailable. Coactive allows you to avoid major setbacks by transferring the metadata and customisations you’ve made to your next model of choice.
Summary: metadata enrichment using multimodal AI boosts search outcomes
The evolution from manual metadata to AI-powered metadata generation represents a fundamental shift in how organizations leverage their content. Modern enterprises need systems that understand content the way humans do – processing multiple types of information simultaneously and efficiently adapting to new requirements.
Ready to see Coactive in action? Request a demo today.